Form Submission: Participation Entry
Research Day Entry
Prediction model for crop productivity in India
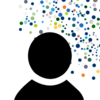
Climate change is aggravating the challenges faced by the agriculture sector. Increasing temperatures, variability in rainfall, and frequency and intensity of extreme weather events are adding to pressure on agriculture systems all over the world. The vulnerability of agriculture to climate change is high in India, where the livelihoods of 600 million people (about 50% of population) depend on agriculture. Climate change is predicted to result in a 4%-26% loss in net farm income towards the end of the century. Such research provided impetus to formulate national climate adaptation policies in India. However, there is no guidance to the policy makers on how these estimates translate into predictions in the immediate future at the disaggregated level. Operating in a highly resource constrained environment, the Indian policy makers often ask which geographies, agriculture seasons, crops and communities are more vulnerable to climate change than others? Which crop insurance policy protects the farmer from the vagaries of climate?
My research engages with some of the above questions by modelling crop productivity of the top-10 crops of India at the district (sub-state) level in India. I have combined government administrative data on crop production at the district level with irrigation data, climate data, soil data, and economic data during the years 1997-2014. I used machine learning methods such as random forests, gradient boosting machine, and neural networks to build a prediction model of crop productivity at the district level in India. Finally, the climate forecasts using Global Climate Models, most suitable for the Indian context are used to predict the crop productivity in the near future at the district level for each crop. The prediction model is expected to assist policy makers and crop insurance companies to provide optimum solutions to farmers through well-targeted or customized programs and products.