Form Submission: Participation Entry
Research Day Entry
Biophysical interactions at the (sub)mesoscale in the pelagic open-ocean
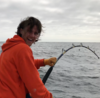
Quantifying the importance of energetic variability at oceanic (sub)mesoscale O(0.1-100km) is inhibited by the fact that current remote sensing technologies are in many cases insufficient for evaluating these features over broad spatiotemporal scales. The known association between (sub)mesoscale features and biological variability suggests the potential for the use of in-situ observations of marine biota to address the limitations of remote sensing methods. This research explores the viability of two such approaches to (sub)mesoscale analysis. First, we show that non-linear dimensionality reduction and consistency training network architectures enable state of the art classification of phytoplankton species composition from imaging flow cytometry data. These data can then be used to train high-performance algorithms for detection of oceanographic variability from spectral measurements of ocean color. Second, we evaluate the use of animal borne CTD tags deployed on pelagic sharks for the observation of subsurface thermal properties of (sub)mesoscale features at broad spatiotemporal scales.